Support Vector Machines (SVM)
Support vector machines (SVMs) are a set of supervised learning methods used for classification, regression and outliers detection.
Classification
SVC
, NuSVC
and LinearSVC
are classes capable of performing binary and multi-class classification on a dataset.
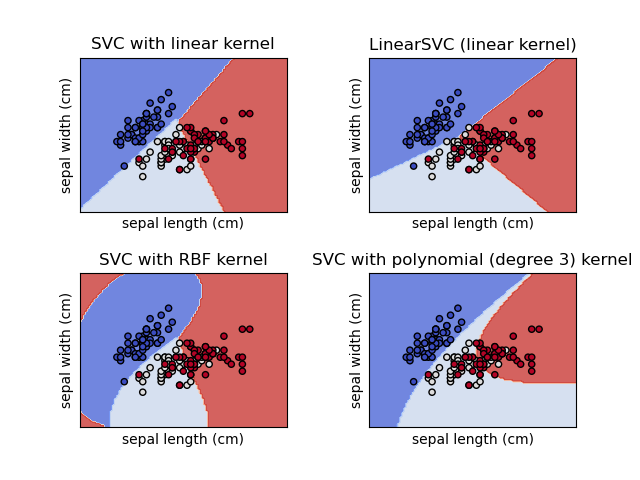
>>> from sklearn import svm
>>> X = [[0, 0], [1, 1]]
>>> y = [0, 1]
>>> clf = svm.SVC()
>>> clf.fit(X, y)
SVC()
After being fitted, the model can then be used to predict new values:
>>> clf.predict([[2., 2.]])
array([1])
SVMs decision function (detailed in the Mathematical formulation) depends on some subset of the training data, called the support vectors. Some properties of these support vectors can be found in attributes support_vectors_
, support_
and n_support_
:
>>> # get support vectors
>>> clf.support_vectors_
array([[0., 0.],
[1., 1.]])
>>> # get indices of support vectors
>>> clf.support_
array([0, 1]...)
>>> # get number of support vectors for each class
>>> clf.n_support_
array([1, 1]...)
Multi-class classification
>>> X = [[0], [1], [2], [3]]
>>> Y = [0, 1, 2, 3]
>>> clf = svm.SVC(decision_function_shape='ovo')
>>> clf.fit(X, Y)
SVC(decision_function_shape='ovo')
>>> dec = clf.decision_function([[1]])
>>> dec.shape[1] # 4 classes: 4*3/2 = 6
6
>>> clf.decision_function_shape = "ovr"
>>> dec = clf.decision_function([[1]])
>>> dec.shape[1] # 4 classes
4
On the other hand, LinearSVC
implements “one-vs-the-rest” multi-class strategy, thus training n_classes
models.
>>> lin_clf = svm.LinearSVC()
>>> lin_clf.fit(X, Y)
LinearSVC()
>>> dec = lin_clf.decision_function([[1]])
>>> dec.shape[1]
4
Regression
>>> from sklearn import svm >>> X = [[0, 0], [2, 2]] >>> y = [0.5, 2.5] >>> regr = svm.SVR() >>> regr.fit(X, y) SVR() >>> regr.predict([[1, 1]]) array([1.5])
No comments
If you have any doubts, Please let me know